During the design of a clinical trial, many biotechs want to substantially reduce the risk of a good new therapy being rejected. Such a risk-reduction is easy to quantify when someone is a statistician. Yet how we communicate this risk, and how non-statisticians understand this reduction of risk, can significantly effect which designs are determined to be desirable.
A biotech recently reached out to Cytel to explore options on optimizing a clinical trial design. After simulating over 150 million patient lives in 1-2 hours, Cytel was able to provide an array of design options that optimized for speed, power and sample size. Yet when aiming to reduce the risk of a faulty conclusion – that is, the risk of a good drug being rejected – the sponsor had to be mindful of a mathematical tradeoff.
When designing and optimizing clinical trials, the target effect is defined as the projected difference between the effect size in the active and control arm. One such effect might be the ‘clinically meaningful effect,’ the effect size at which it is possible to determine that one arm has superior clinical effect than another.
Yet ‘clinically meaningful effects’ are usually much smaller than desirable target effects. For the small biotech, the clinically meaningful effect that was in their reference design (that is, the design to be optimized) generated about 50% of the power of the target effect.
When increasing the power of a study design, across several different possible target effects, a smaller increase in absolute power in an optimistic effect can reduce the risk of a faulty conclusion by a greater percentage than a greater increase in a pessimistic effect. So for example, a 4% increase in absolute power assuming an optimistic target effect size translated to a nearly 30% reduction in risk; while a 7% increase in actual power for a clinically meaningful effect translated to a 13% reduction in risk.
Very simply, this is because for a given sample size, a pessimistic target effect begins with lower anticipated power and a higher chance of being underpowered. Yet when a clinical trial sponsor hears a 30% risk reduction, it is the duty of a statistician to explain what this means.
One way to do so is through a clear and compelling visual, as found in our new case study which delivered a 4% to 8% increase in absolute power across three target effects. Read the study to learn more:
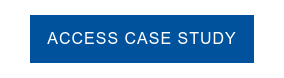
About the Author of Blog:
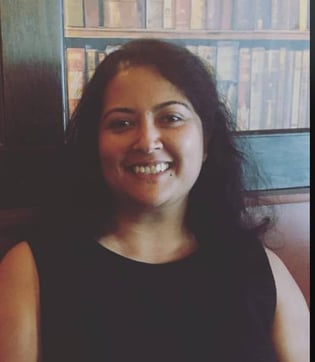
Dr. Esha Senchaudhuri is a research and communications specialist, committed to helping scholars and scientists translate their research findings to public and private sector executives. At Cytel Esha leads content strategy and content production across the company's five business units. She received a doctorate from the London School of Economics in philosophy, and is a former early-career policy fellow of the American Academy of Arts and Sciences. She has taught medical ethics at the Harvard School of Public Health (TH Chan School), and sits on the Steering Committee of the Society for Women in Philosophy's Eastern Division, which is responsible for awarding the Distinguished Woman in Philosophy Award.