Sample Size Re-Estimation for Rare Disease Clinical Trials
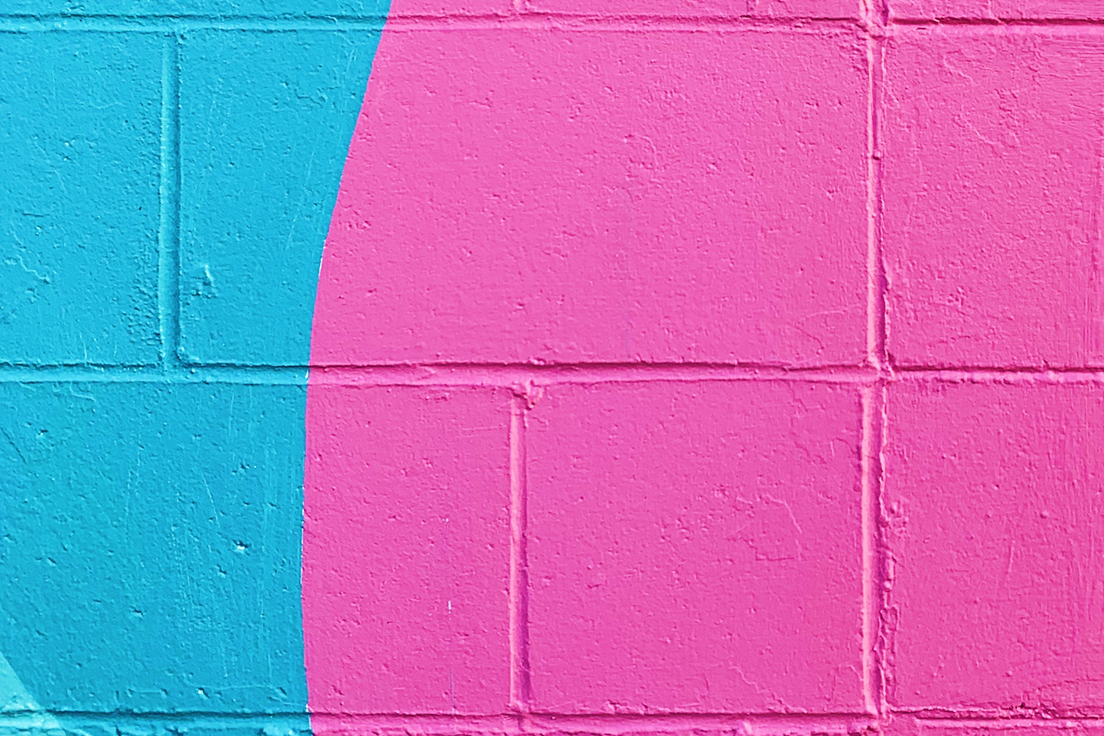
Written by Boaz N. Adler, MPA, Director, Global Product Engagement, and Valeria Mazzanti, MPH, Associate Director, Customer Success
In our previous post, we provided an overview of some of the challenges in studying rare disease therapies, as well as some of the statistical methods and design types that can be deployed to mitigate these challenges. Here, we focus more specifically on one of these methods: sample size re-estimation, and its promise to help control both risk and costs of rare disease studies.
The true underlying treatment effect and study design
We can never be too sure of the true underlying treatment effect for the therapy that we are evaluating in a clinical trial, as there is much variability in how therapies affect individual patients. That said, when designing a clinical trial, we always start with an estimate in mind, typically taken from previous studies or from literature reviews. This estimate allows statisticians to determine how many subjects they must enroll into the study to ensure they will be able to collect enough evidence to suggest their treatment works well. However, if the number of subjects computed is based off of an incorrect estimate, the statistical power of the study plummets and the overall findings of the study cannot be used in support of advancing the treatment to market.
Although enrolling as many subjects as possible into a trial is attractive in the context of statistical power, it is unfortunately a very expensive practice, and it may be unethical to expose more patients than necessary to a clinical procedure or treatment. Therefore, statisticians must identify the “sweet spot” between enrolling enough subjects to prove the treatment under investigation works, but without surpassing the minimum number of patients needed and budget constraints.
What is sample size re-estimation?
Sample size re-estimation is a powerful risk-mitigation tool that sponsors can deploy to reduce investing in failing studies due to uncertain or unrealistic treatment effect expectations.
In adaptive designs, one purpose of an interim analysis is to determine whether to stop the study early for efficacy, stop for futility, or continue the study, based on interim data collected up to the analysis time. The addition of sample size re-estimation methodology simply means adding a few more options to the decision matrix presented at this interim look. In addition to the three existing options — ending the study early for efficacy or futility, or continuing the study as planned — an interim analysis can lead to three additional “zones” or categories: unfavorable, promising, and favorable zones that lead to different trial continuation decisions. The decision matrix at the interim analysis is as follows:
Zone/Category |
Observation |
Decision |
Futility |
Observed treatment effect is not better than control. |
Stop the study early and declare futility. |
Unfavorable |
Observed treatment effect is showing some improvement over control, but not sufficient to merit additional investment. |
Continue study with originally planned sample size. |
Promising |
Observed treatment is close to that which was expected/planned, within a promising range of effect. |
Continue the study with an increased sample size according to pre-specified plan to increase the overall power or probability of winning of your study. |
Favorable |
Observed treatment is as expected, and there is little concern about the study achieving its goals with the originally planned sample size. |
Continue the study with originally planned sample size. |
Efficacy |
Observed treatment effect is overwhelmingly in favor of the treatment. |
Stop the study early and declare efficacy. |
The addition of the favorable, unfavorable, and promising categories into the decision matrix allows a less risky approach to both overall trial execution, and investment in additional study subjects based on true underlying data observed in the study.
What determines the amount of additional investment or sample size in the design?
As noted above, the magnitude of the increase in sample size in a Sample Size Re-Estimation design must be pre-specified in the study protocol and approved by the regulator before study launch. This magnitude is typically expressed as a multiplier over the original study sample size and is based on a worst-effect scenario that the study team may agree is still clinically meaningful and financially viable for the product under development. The additional, lower treatment effect assumption is then often used to calculate the boundaries of the five different zones described in the decision matrix.
Final takeaways
Sample Size Re-Estimation is a powerful tool to both gatekeep investment in clinical development and help reduce the overall required sample size in studies with uncertain treatment effects. Study teams can then commit to higher resource use, and agree to higher development costs, once they have some guarantee that the trial is leading to a potentially positive result.
Interested in learning more about strategies to mitigate the challenge of limited patient populations in rare disease studies? In their upcoming webinar, Boaz Adler and Valeria Mazzanti will discuss this topic and share an example of sample-size re-estimation in a Phase III Sjogren's Disease study. Click below to register. Can’t make the time? Register today and watch it on-demand after the event:
About Boaz N. Adler
Boaz Adler is Director of Global Product Engagement at Cytel. He has served as a Solutions Consultant and Analyst for Life Sciences companies and Health-Tech organizations for over a decade. Boaz’s interests are focused on tech and novel services innovations that contribute to more coherent and robust evidence generation across the drug development cycle. At Cytel, Boaz enhances the connection between Cytel’s software development team and its clients and supports clients in clinical trial optimization projects using Cytel’s cutting-edge technology.
About Valeria Mazzanti
Valeria Mazzanti is Associate Director of Customer Success at Cytel. She is an expert in adaptive clinical trial design methodology and software, including cutting-edge and industry standards such as Solara®, East®, and EnForeSys®. Prior to joining Cytel, Valeria worked in several different academic research laboratories, and has extensive teaching experience at prestigious universities. Valeria completed a Master of Public Health degree specialized in Biostatistics at Columbia University in New York and a Bachelor of Science degree in Behavioral Neuroscience at UCLA.
Read more from Perspectives on Enquiry and Evidence:
Sorry no results please clear the filters and try again
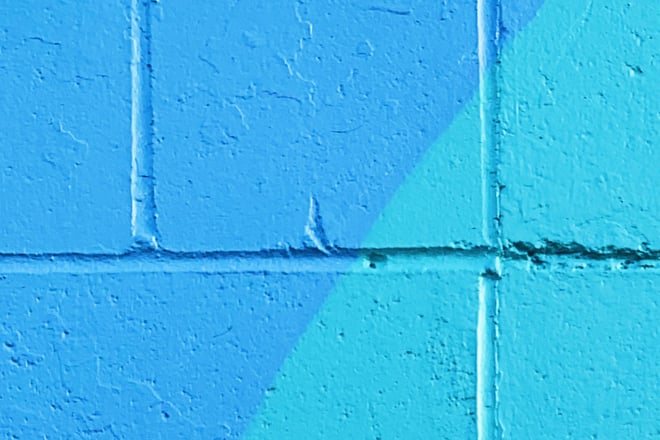
Strategies to Overcome Limited Patient Population Challenges in Rare Disease Studies
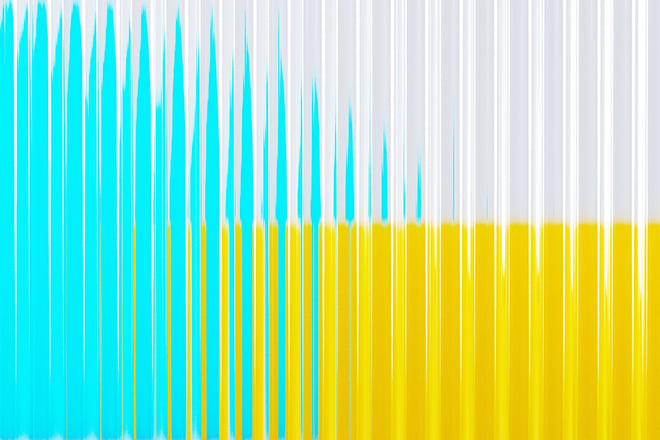
How to Ensure Your Adaptive Trial Is Appropriate for Regulatory Submission
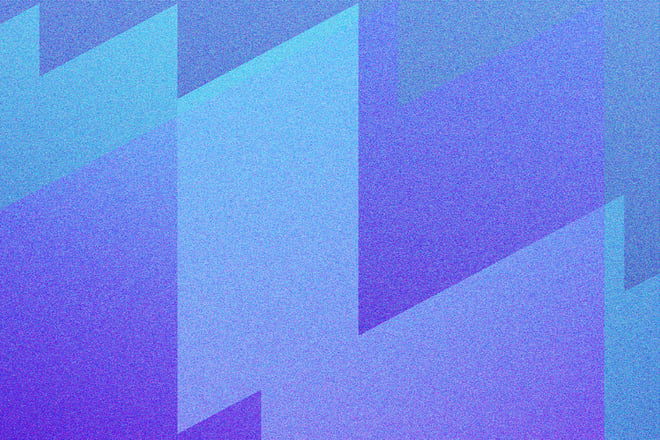
Optimizing Early Clinical Development Strategy