How to Use Historical Data to Mitigate Clinical Trial Risk
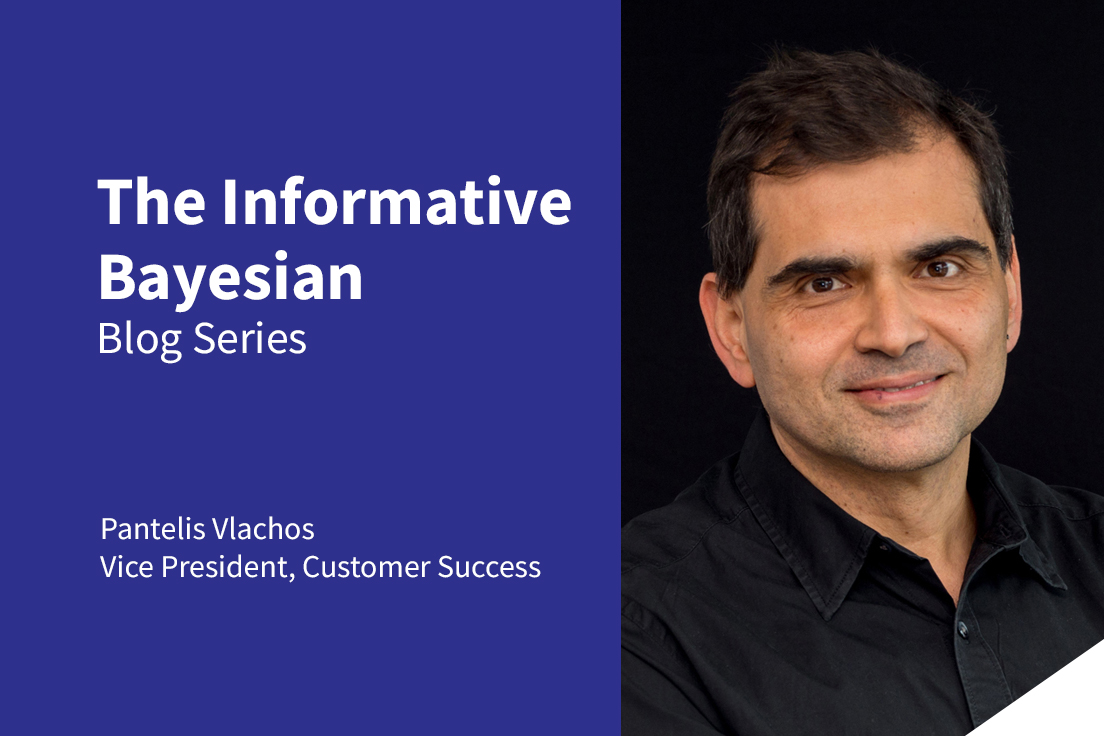
For over a decade, the number one reason cited for trial discontinuity has been challenges associated with recruitment and enrollment. Oftentimes this occurs after millions of dollars have already been invested in opening clinical trial sites and implementing the infrastructure for data collection and management. An even higher opportunity cost arises, if and when the trial could have successfully completed with resulting regulatory approval.
Once we incorporate historical data into the process of recruitment forecasting, new possibilities arise that have implications for both enrollment and clinical trial design. While the novel uses of historical data are still evolving, and quantitative expertise is necessary to adopt these methods with precision, it is also necessary to begin using these methods widely to decrease unnecessarily wasted opportunities that can drive up the costs of drug development.
Introduction
As noted in a previous blog , the foremost hurdle to meeting enrollment targets appears to be securing reliable processes of forecasting enrollment. Currently the process is riddled with oversimplified assumptions that ensure inaccurate projections. In response, Cytel created a software tool that enables sponsors to ask for more nuanced details about site selection and enrollment hypotheses by using a quantitatively rigorous forecasting algorithm.
Instead of incorporating linear assumptions, the forecasting tool provided by Cytel enables sponsors to investigate more complex scenarios. For example, a traditional forecasting method might have assumed that Trial Site A recruits 30 patients per week, because on average this appears to be true. We know, though, that there is variation from week to week. As a result, when trying to determine whether Trial Site A can recruit 100 patients in the next month, there might be some uncertainty.
A more fruitful line of investigation might be to ask whether there is at least an 80% chance that Trial Site A will recruit 100 patients in the next month. If we realize that Trial Sites A and B each have an 80% chance of recruiting 100 patients in the next month, but that Trial Site C for some reason has a 60% chance of securing 300 patients in the month following, we can adjust both our resources as well as our timelines to align with the given risks. Sponsors will be able to ascertain, for example, whether it is realistic the try to recruit 300 patients in 6-8 weeks, and what types of investment are needed in order to do so.
Patient Enrollment & Clinical Trial Design
One question that naturally arises is whether clinical trial design can anticipate and mitigate risks associated with enrollment. For example, if choosing between a group sequential design or a traditional design, or between designs which have significantly different trial lengths or different numbers of arms, would it not be helpful to know what reasonable enrollment strategies exist for each design? Some designs might save cost and power, but be less feasible. Other designs might avoid some of the pitfalls of patient recruitment that a clinical operations team might see, but which a biostatistician might not.
Scientists at Cytel have argued that for superior strategic and technical insight, it is important for these functional units to begin coordinating much earlier in the design process. Our clients testify again and again to the insights such collaboration provides, but have also witnessed how frustrating it is to begin these conversations. One of the many capabilities of Cytel’s enterprise level platform SolaraTM, is to simplify this process by channeling data and computation to greater visibility for all involved.
Data: A Key Ingredient to Clinical Trial Strategy
Opening up such channels of strategic learning has also opened up opportunities to bring novel solutions to age old questions. Central to this is the uses of historical data for both clinical trial design and feasibility studies.
In an upcoming Cytel webinar, I will reveal how to use historical data to test recruitment scenarios and predict milestones for enrollment, and show how to use these techniques when confronted with issues like delayed treatment effect and dropout rates. I will also shed light on the important question of how trial design can ameliorate the ripple effects of uncertainty in feasibility studies.
Click below to learn more:
About Pantelis Vlachos
Pantelis is Vice President, Customer Success for Cytel, Inc. based in Geneva. He joined the company in January 2013. Before that, he was a Principal Biostatistician at Merck Serono as well as a Professor of Statistics at Carnegie Mellon University for 12 years. His research interests lie in the area of adaptive designs, mainly from a Bayesian perspective, as well as hierarchical model testing and checking although his secret passion is Text Mining. He has served as Managing Editor of the journal “Bayesian Analysis” as well as editorial boards of several other journals and online statistical data and software archives.