How to leverage model-informed drug development for rare diseases
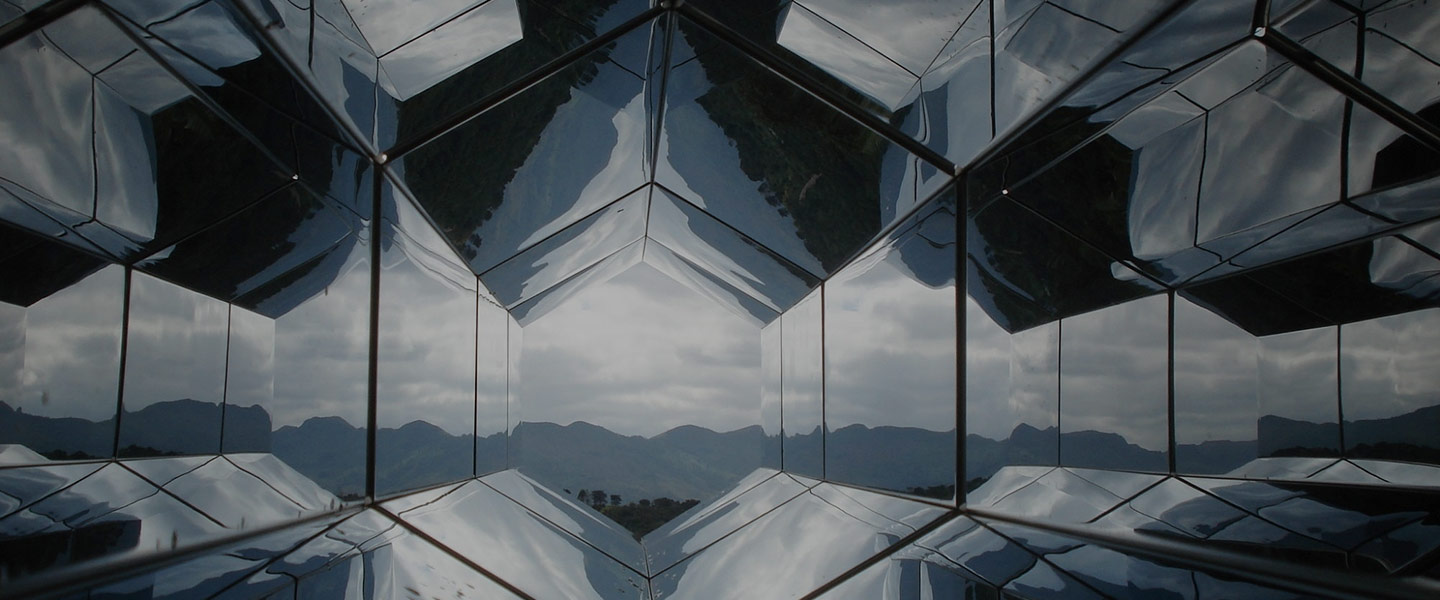
While there is a plethora of rare diseases, some 7000 diseases and counting, one needs to consider the statutory definitions of rare disease per region as there is considerable variability in how such diseases are defined in the public domain. For example, in the United States, a rare disease is defined as a condition that affects fewer than 200,000 people in the US. This definition was created by Congress in the Orphan Drug Act of 1983. In the European Union, by contrast, a disease is defined as rare when it affects fewer than 1 in 2,000 people. These definitions vary amongst geographic regions. To complicate things further, in resource-poor regions the term “neglected disease” is applied to a handful of tropical infections that lack adequate incentives for drug development or mechanisms to make existing treatments available.
The Orphan Drug Act created financial incentives to encourage companies to develop new drugs for rare diseases. There are quite significant incentives granted under this act, including but not limited to market exclusivity, tax benefits, and priority review vouchers. Rare diseases often qualify for fast track regulatory processes within the US, including accelerated approval and priority reviews. There are similar benefits afforded within the existing laws within other regions of the world.
Needless to say, such opportunities for rapid accelerated development are a fuel for disruptive innovation because of the rather specialized nature of the target patient population, including access of those patients for clinical trials. Conventional drug development stops at its wheels where a rare disease development program is considered.
There are 3 key considerations that relate to designing a clinical trial for rare diseases:
- Enriching the trials with biomarkers
- Ensuring pharmacokinetic characterization with a choice of optimal sampling designs
- Out of the box clinical trial designs
We address each one of them below:
1. Enriching the trials with biomarkers
There are many challenges inherent in rare diseases clinical research. These challenges include scarcity of the patient population, uncertainties in duration of disease progression and natural history, as well as a general imperfect understanding of the disease pathophysiology. A vast majority of rare diseases are without validated or surrogate endpoints. These issues collectively complicate the selection of endpoints and biomarkers. The challenges have resulted in a greater emphasis on biomarkers either through company initiatives or through public-private partnerships.
The rational use of biomarkers offers a vehicle to accelerate the development of drugs for rare diseases. Not only do biomarkers assist in the selection of doses, but they may also be used to indicate responsiveness to treatment. This is quite important in rare diseases since a vast majority of diseases have a genetic validation. Qualification of biomarkers might also reduce the number of subjects in a trial. Regulatory health authorities typically acknowledge these challenges and would often accept unvalidated biomarkers if there is a weight of evidence and sound scientific rationale supporting those choices.
Let us consider Huntington’s disease (HD) as an example. This is a progressive neurodegenerative disorder that has sound genetic validation in that there is a polymorphic trinucleotide (CAG) repeat expansion in the HD gene (HTT) gene. (1,2) Early research into biomarkers for HD relied on the use of magnetic resonance imaging. For example, changes in striatal volume using MRI could be considered as a biomarker. (2) More non-invasive markers have been the subject of recent interrogations in the field. The challenge however is that HTT is expressed outside the brain. There was considerable uncertainty whether the pathophysiology of Huntington’s disease is only restricted to the brain or to peripheral systems. Symptomology simulating these peripheral systems has been noted in patients presenting themselves with HD including abnormal glucose homeostasis. Of the many biomarkers assessed for HD including inflammatory biomarkers such as c-reactive protein or interleukins, scientific questions arise whether these markers are of more relevance and associated with general motor and cognitive impairment. A recent effort showed that whereas plasma IL-6 did not relate with HD symptoms, salivary IL-6 did. (1) Their work highlights the relevance of a chosen biomarker, where it is sampled from, and how it relates to clinical manifestations of disease and the challenges associated with these considerations.
2. Ensuring pharmacokinetic characterization with a choice of optimal sampling designs
In many rare diseases, there are no known validated biomarkers or surrogate endpoints. In their absence, it becomes important to ensure pharmacokinetics of investigational new molecules and biologics are well characterized. The scarcity of the patient population does present significant logistical burden to trial sites often hindering the sampling and bioanalytical processing considerations inherent in these investigations. However, since characterization of PK and therefore PK/PD can facilitate meaningful conversations around covariates of significance for both intrinsic and extrinsic factors as well as posology and package insert negotiations, ensuring PK is optimally collected remains a priority for rare disease research. Due to the scarcity of the population, regulators often expect PK sampling to be accomplished in all subjects enrolled in these clinical trials.
It is important to note that while negotiating the balance between sampling burden and conduct of the trial, sufficient PK sampling is needed to yield acceptable parameter estimation. This is because there is clear evidence that the way we sample can affect how these parameters are estimated and could lead to errors in estimation that may render the whole exercise less meaningful. (3) There are approaches that can optimally design these PK sampling windows which have now been in practice in population pharmacokinetics experiments. (4) In addition, such optimal sampling windows may be more informative than discrete sampling time points while adding flexibility to timing of sample collection. In fact, the use of sampling windows vs fixed time points also has been argued to reduce structural and statistical model parameter misspecifications. (5) For a good review of the optimization of PK sampling using D-optimality in such studies, refer to the work of Obungberno and Aarons.(4)
3. Out of the box clinical trial designs
By definition, a rare disease has a small population of patients hence, it is difficult if not impossible to obtain a large enough sample of patients for large scale pivotal clinical trials. Traditional endpoints like proportion of "responders" require sample sizes that may be infeasible due to the scarcity of patients. Some diseases are multifaceted, involving different disabilities, making endpoint choices difficult. For example, some patients with rare muscular-skeletal diseases have strength loss and physical function performance difficulties involving different functions and muscle groups. This makes use of physical function tests varied depending on patient. Other patients have more advanced disease and are immobile and not able to perform physical function tests. Disease rareness makes confining a trial to a homogeneous sub-population infeasible; hence, composite endpoints may be needed to assess an available heterogeneous patient sample for a clinical trial. These difficulties require creative statistical approaches to design definitive clinical trials for rare diseases. Therefore, adaptive trial designs, Bayesian methods, and N-of-1 trials can be especially useful for rare diseases to maintain necessary statistical precision with smaller sample sizes than traditional large-scale non-adaptive trial designs based on frequentist statistical methods.
Adaptive trial designs (6) use accumulating data from the patients in the trial to modify aspects of the trial design during the trial to make the trial more efficient - i.e., minimize numbers of patients required, minimize duration of the trial, or improve statistical precision of the trial (e.g., study power) for a given sample size. The potential modifications need to be pre-planned at the trial design stage and their performance characteristics documented prior to trial initiation. Group sequential designs use interim analyses to stop the trial for an early positive or futility conclusion. If a trial is unlikely to yield positive results and can be stopped early, the interim data can be used to inform on reason for trial failure and inform on a better design of the next trial. Obviously, an early positive result yields an earlier regulatory agency filing to make the test treatment available to patients earlier than would have been achieved by a traditional non-adaptive design. Sample size re-estimation can be added to an interim analysis to modify the planned final sample size, in case interim analysis results indicate that the planned sample size is inadequate to maintain planned statistical power, but desired power could be achieved by increasing the sample size to a pre-planned maximum.
Existing information about current standard-of-care for the rare disease of interest can be leveraged to optimize trial design via a one-treatment design or a Bayesian analysis of a treatment-versus-control 2-group design. If sufficient information exists to document the maximum magnitude of effect on the primary endpoint, then only the test treatment need be studied and its magnitude of response can be compared to that maximum magnitude of effect via a 1-sample statistical test. That maximum magnitude of effect against which the test treatment is compared needs to be well-accepted by the scientific and regulatory agency communities.
If a control group is needed, and if a source of primary endpoint data external to the trial is available from the same population that is sampled in the trial, then it can be used as an informative prior for a Bayesian analysis (7) to compare the test treatment to the combined control group consisting of the external control treatment data and the control treatment data from the trial. This can lower the trial's control group sample size required for adequate statistical precision.
A key challenge for use of one-group trials and 2-group trials with Bayesian informative priors is demonstrating that the population that generated the external control data is the same as, or sufficiently similar to, that of the control group in the trial. If individual patient data are available from the external controls, then matching on key factors can be used to select the particular external controls that are used in the trial's analysis. Otherwise, summary statistics on key factors would be needed to match the external control source to the control group in the trial.
Finally, N-of-1 trial design (8) can be considered if treatment effect can be observed rapidly enough to afford a sequence of treatment periods within the same patient. For example, if 2 control periods and 2 treatment periods each yield an observation of the primary endpoint, this could be sufficient to yield a statistical conclusion of treatment efficacy within that one patient. Obviously, if more treatment periods are feasible within a patient, then more precise statistical precision can be achieved than that from 4 treatment periods.
In summary, rare disease drug development is at an interesting crossroad of opportunity and collective engagement of multiple stakeholder groups. With the advancement of the 21st Century Cures Act, there is a regulatory path of leveraging real-world evidence for drug approvals. The continued emphasis of model-informed drug development and the optimal use of the “Lew Sheiner’s learn and confirm” paradigm will only serve to ensure maximal probability of success of these development programs.
References:
1. Corey-Bloom J., Fischer R.S., Kim A., Snell C., Parkin G.M., Granger D.A., Granger S.W., Thomas E.A. Levels of Interleukin-6 in Saliva, but Not Plasma, Correlate with Clinical Metrics in Huntington’s Disease Patients and Healthy Control Subjects. Int. J. Mol. Sci. 2020;21:6363. doi: 10.3390/ijms21176363.
2. Aylward, E. H. 2007. Change in MRI striatal volumes as a biomarker in preclinical Huntington’s disease. Brain Research Bulletin 72(2-3):152-158.
3. al Banna MK, Kelman AW,Whiting B (1990) Experimental design and efficient parameter estimation in population pharmacokinetics. J Pharmacokinet Biopharm 18:347–360.
4. Obungbenro K and Aarons L. Optimisation of sampling windows design for population pharmacokinetic experiments. J Pharmacokinet Pharmacodyn (2008) 35:465–482
5. Green B, Duffull SB (2003) Prospective evaluation of a D-optimal designed population pharmacokinetic study. J Pharmacokinet Pharmacodyn 30:145–161.
6. Bornkamp B et al. Innovative Approaches for Designing and Analyzing Adaptive Dose-Ranging Trials. Journal of Biopharmaceutical Statistics 2007;17:6, 965 — 995.
7. Berry DA. Bayesian clinical trials. Nature Reviews Drug Discovery 2006; 5: 27-36.
8. Lillie EO et al. The n-of-1 clinical trial: the ultimate strategy for individualizing medicine? Per Med. 2011 March ; 8(2): 161–173. 2011
Suggested readings:
- Adaptive Designs for Clinical Trials of Drugs and Biologics (Nov 2019)
- Guidance for the Use of Bayesian Statistics in Medical Device Clinical Trials (Feb 2010)
- Rare Diseases: Common Issues in Drug Development (Aug 2015)
- Report: Complex Issues in Developing Drugs and Biological Products for Rare Diseases and accelerating the Development of Therapies for Pediatric Rare Diseases Including Strategic Plan: Accelerating the Development of Therapies for Pediatric Rare Diseases (July 2014)
About the Authors of Blog:
Rajesh Krishna
Rajesh Krishna, PhD is a Senior Director in Integrated Drug Development at Certara Strategic Consulting. Rajesh came to Certara after a rich 20+ years of pharmaceutical industry experience in translational and clinical development at Merck, Sanofi (legacy Aventis), and Bristol-Myers Squibb. Rajesh has been involved in the development of a variety of therapeutic modalities (e.g., oral small molecules, long acting injectables and implants, biologics, and vaccines) and therapeutic areas (e.g., infectious diseases, immunology, oncology, neurosciences, cardiovascular and metabolic diseases). While at Merck, he also spent a year at the MSD Translational Medicine Research Center in Singapore, where he was the early clinical lead with a focus on digital medicine and regional tropical diseases.
James "Jim" Bolognese
James Bolognese is a senior research fellow at Cytel and is a guest contributor to this blog. He has a broad statistical experience in clinical research spanning the entire drug development lifecycle, including pre-clinical, clinical pharmacology, Phase 2, late-stage clinical development and outcomes trials in both large-scale pharmaceutical and biotech companies. Prior to joining Cytel in 2008, James was Senior Director of Biostatistics at Merck in Rahway, NJ, USA, which he joined in 1976 after obtaining his Masters degree.